Conceptual Framework
Curator Systems
Sub. Question 1: Are the recommendations from the curator system more acceptable by users?
The introduction of curator systems in general recommendation models could potentially influence the users’ acceptance rates of related suggestions due to certain attributes of the framework. There are features of a recommendation system that integrates curators that would boost its appeal and therefore recommendations given. Specifically, we consider the following: curator system vs general recommendation, perception of trust, type of curator and transparency. Curator based systems would integrate sophisticated algorithms which make theoretical considerations for the users want of the same content. Essentially, they create social context for interactions, given that humans associate with things based on their perceived meaning (Chipp et al., 2018). A general recommendation system on YouTube for example, would suggest a link with no additional information, while a curator system not only offers a link of curated content but also ideas on what services or products to purchase.
There are many factors that influence users’ acceptance rate of underlying recommendation systems with its architecture being key, with regards to either collaborative filtering or contentbased methods used to determine likely user preferences. Curator systems would offer enhanced integration of users’ preferences and a more personal touch which directly influences trust levels of resulting recommendations. This takes form in one of three approaches, where the first uses social networks and browsing history to create a crowd curated environment with users as the curators. Alternatively, the basis of determination could be user information collected overtime that is ultimately managed by them or a combination of user-based and traditional collaborative filtering to improve efficiency of a recommendation system (Dragovic, Azpiazu & Pera, 2018). Organizations are exploring the additional value that infusing a more personal touch into their content and marketing strategies yields on their revenue generation, especially in more service-based industries. Implementation of curators into general recommendation systems on a wider scale would provide tangible evidence of their effect on acceptance rate through positively affecting purchase intention and related features.
Consumers that use a curator system have more control over the suggested content, with the premise being their personal preferences, essentially curator-based recommendation systems do not take on the role of curator. This is part of their appeal, as consumers in the current market are overloaded with information and may question whether advertisements are based on their tastes or organizations with a wide advertisement reach. The opinions of community users make suggested services or items feel more authentic and narrows down selection to a set of relevant items from an extensive set of choices. Part of the curator system’s viability is based on the algorithm applied in making predictions, with flexibility based on feature attributes enabling tailoring to better suit the dataset and analysis required. Recommender systems success metrics are dependent on these measures, where higher model accuracy makes it more likely that users will accept resulting recommendations (Herlocker et al., 2004). Essentially, curator systems are built on consumer behavior, with the added benefit of perceived expertise authenticating the process and suggestions. We therefore expect the following:
H1: Customers who use a curator system would have higher acceptance rate of its recommendations offered than the general recommendation system.
Trust
Sub. Question 2: Will the use of curator enhance the trustworthiness of the automatic recommendation system?
Curator systems could help to alter the users’ perceptions of trust, as they bridge a platform of engagement essentially mediating a relationship between the type of systems and willingness of accepting recommendations. This is modelled after a mediation hypothesis theory, which would explain the effect that trust perceptions have on user acceptance rates through examining the effect that an underlying variable would have on the process. Essentially, the introduction of a curator in traditional recommendation systems is expected to influences users perceptions of trust in the preliminary stages of the process of mediating trust. This is because choice is driven by a calculated decision based on perceived advantages, where a trustor is more likely to cooperate after reasons to do so have been established (Komiak & Benbasat, 2006). Consequently, curator systems application is expected to positively influence recommendation perceptions from users, as it establishes a strong cognitive trust basis, which is elemental for emotional trust connections.
Emotional trust helps to bridge a trust gap that cognitive processes cannot due to the nature of e-commerce landscapes, as it helps to alleviate concerns such as uncertainty about the attributes and traits of the vendors they interact with. Given how difficult it is to establish trust on virtual platforms, curator systems would bridge this gap as they integrate the more personal aspects of social media with business elements of e-commerce. They would therefore help in positive perceptions of recommendations by providing relevant yet personal interactions with and suggestions to users. Trust helps consumers translate their feelings into actions, due to the feelings of security associated with the object at hand which allows steps to be taken despite the risks involved (McKnight, Choudhury & Kacmar, 2002). Curator based systems are therefore expected to make elemental differences in preliminary interactions between users and vendors or e-commerce sites, that positively influences their decisions on whether to use the services in future. Given the inherent risk associated with online shopping platforms such as privacy, quality restriction and intention of advertisement, the role of trust is more prevalent than ever.
Influencers have been found to be more believable than traditional automated systems due to the proven integration of human complements into the process, and would form part of the curator eco-system. Curator systems are expected to positively affected purchase intentions as consumers are more likely to positively perceive attributes such as benevolence, reputation and privacy concerns present in traditional systems. Research on related issues support the notions, with close to 60% of purchase intention being linked to trust (Oliveira et al., 2017). The introduction of curator systems incorporates not only the users’ preferences but an eco-system of similar beliefs managed by a trusted platform, which positively influences the recommendations finally presented. The risks and concerns present in e-commerce platforms are especially challenging to overcome due to the lack of personal interaction with consumer, CS would significantly help. In order to examine this effect, the mediator role of trust needs to be first tested:
H2: Trust mediates the relationship between willingness to accept the recommendations and the types of system used.
Curator Types
Sub. Question 3: Will the types of curator affect users’ perception of trustworthiness of the recommendations and how?
The type of curator involved with the process is fundamental in establishing a robust framework which could either generate substantial and positive engagement and conversion or underwhelming results. This is the result of the social relationship designed and ultimately generated by curators, who in the modern business age are in charge various steps such as designing delivery systems from technical perspectives, or managing the content released in the market through influencer strategies for example. Ultimately, even in instances where suggestions are purely digital, consumers still apply human social rules and expectations to computers (Qiu & Benbasat, 2009). In automated models, the informational disparity humanness of the system is a key factor in enhancing the quality of recommendation systems and ultimately acceptance rates. Consequently, the type of curator system implemented becomes elemental in determining the level of acceptance resulting suggestions elicit from consumers with complaints of innately robotic capabilities for exampling generating low engagement.
Curator Popularity
We present curator popularity as a fundamental factor in influencing purchase intention given that visibility is as important in the current business environment. The popularity of curator system and related personnel is important in generating a high acceptance rate among users with there being tremendous power in numbers. The trendier items and services suggested are, and the person making these recommendations are the more likely that consumers purchase suggested items. Consequently, the reach that related systems have should be enhanced through well deliberated and designed systems. Google is an example of a curator that manages its information in similar fashions, where the most popular content is fashioned rather than the most relevant, which has resulted in the organization having the most inbound links. Certain curator suggestions are likely to foster more support owing to noted market popularity and acceptability, with book sites like Goodreads for example basing recommendations on activity generated by underling products (Wang, Zhu & Caverlee, 2020). The popularity of curators and the effect on acceptance is an interesting relationship, with high engagement and acceptance rates feeding the following expectations:
H3a: The popularity of curators is positively related to user’s intention of accepting the RS’s/CS’s recommendations.
Curator Expertise
The factor of curator expertise also affects acceptance rates where we suggest that the more provenly established a curator or related systems are, the more acceptable its recommendations are to users. The users are more sensitive to misinformation and more likely to withdraw support from connected organizations, which makes how knowledgeable curators and related systems are key in generating positive engagements. Consequently, preliminary specialization should be integrated into the framework, with a proven level of expertise expected to generate more trust from users with regards to recommendations. The source of recommendations is a fundamental factor in determining whether or not users trust information from certain sources, with a distinction of personal or impersonal ties aiding users to make decisions. This is because the sources lead to personal or impersonal recommendations which are either helpful or detrimental to acceptance (Kunkel et al., 2018). Furthermore, consumers are more interested than ever on ethics and the veracity of information disseminated by organizations. Consequently, curator-based systems that are better received and more popular in the market are often backed by verifiable source and a certain level of expertise. Thus, we expect the following:
H3b: The expertise of curators is positively related to user’s intention of accepting the RS’s/CS’s recommendations.
Transparency of the Recommending Process
Sub. Question 4: Will the transparency of the recommending process of the system increase user’s perception of its credibility, therefore also enhance users’ willingness to accepting the recommendations?
How transparent a recommendation or curator system is could either positively or negatively affect how users perceive and therefore react to suggestions. The issue of transparency of recommendation systems is gaining traction, with users being more apprehensive on interacting with certain organizations and platforms due to privacy concerns. This is due to the significant knowledge gap on how organizations collected and utilize information through interactions on digital platforms. This breeds grounds for related developments as a means of fostering trust and long-term engagement in recommendations and with related curator systems (Vorm & Miller, 2018). Organizations have discovered links between the performative aspects of similar structures and perceived trust, fostered through activities that enable end users to better understand the processes guiding the organization.
Steps such as designing and implementing system-generated response programs that justify why certain recommendations have been made provides users with the logic behind suggestions and increase the likelihood of acceptance. The results mirror similar studies that show system explanations increases trust levels, consumer understanding, enhances better detection and error handling and calibrates a healthy degree of reliance on predictive aids. Transparency is further enhanced through providing certain types of information to users as an alternative to justifications as this requires robust analytical structures, with issues of subjective definition of a good explanation presenting additional challenges. Examples of transparency models to consider include but are not limited to actor model which reveal the source of information and transparency depth pyramids which explore data, process and policy transparency foundations (Hosseini et al., 2017). Through integrating these models into recommendation and curator systems, organizations enhance the quality of relationships fostered with users and therefore increase likelihood of positive reception.
The current business environment is also fraught with social activism, with consumers examining the underlying principles that govern the organization, with apprehension of transparency being a troubling sign. Essentially, organizations enhance the data exchange process through giving the users more control in form of transparency, with consumers being relatively worried about how their personal data is used with 97% of participants of a survey citing concerns on misuse of personal data (HBR, 2015). Transparency is therefore important for not only establishing trust but also rationalizing the product or service quality that users receive. A release of different sources of information used to guide curator or recommendationbased systems could directly influence whether or not consumers accept suggestions. Selfreported data is for example valued less than digital exhaust whereas profiling data is the most valuable. While increased transparency might leave the organization vulnerable, they may also have a positive impact on overall engagement and results, therefore leading to the following expectations:
H4: Higher transparency of recommendation process systems strengthens the relationship between perception of trust and the willingness to accept recommendations
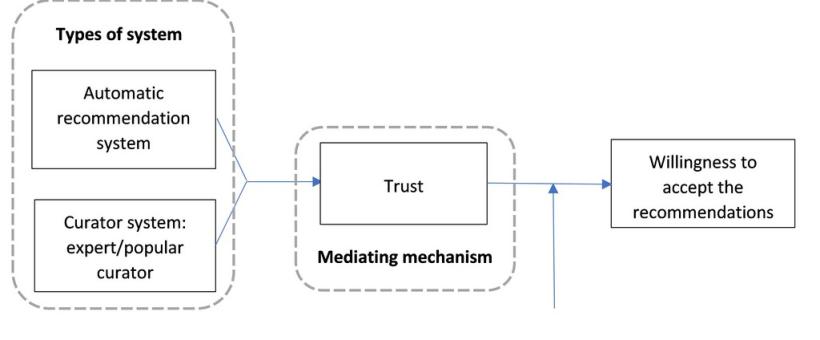
As the visual representation of the model (picture 2) shows, this research investigates how different types of recommendation system could increase users’ perceived trust, which ultimately influence users’ willingness of accepting the recommendations. Then it will also investigate into one important factor that might affect the relationship between trust and recommendation acceptance, transparency of the recommendation process.