Literature Review
Trust lacking in an online environment
Since the evolution of e-commerce, purchasing behavior, information acquisition, and transaction interactions of people have been changed in terms of web applications. The notable examples of this emerging trend are weblogs, Friend-of-a-Friend files, wikis, and social interactions sites. Research has found trust as an important factor that influences consumer behavior (Kharel, 2018). Most consumers perceive online shopping more risky than traditional shopping due to a lack of physical interaction and physical clues(Gustavsson & Johansson, 2006). They are not able to physically view or touch a product before making any purchase, which emphasizes the distance between buyers and products, and the distance between the expected and actual received product (Tatiana et al., 2020). Due to the lack of physical interaction, anonymity, and distance, the potential risk in e-commerce is higher. The majority of the consumers appreciate the real-life experience of touching and trying items. Therefore, it is critical to study online trust to understand why consumers do or do not involve in e-commerce activities(Bach, da Silva, Souza, Kudlawicz-Franco, & da Veiga, 2020).
Furthermore, the monitoring of E-vendor behavior is difficult (Hamelink, 2001), as it gives vendors a higher ability to be opportunistic and take advantage of online consumers (Gefen et al., 2003), resulting in higher consumer perceived risk towards brand and difficulty to build credibility and trust of the brand. The vast information asymmetries in online information bring consumers uncertainty towards vendors’ intentions. It is hard for consumers to determine whether the vendor would acting in their best interest (Elizabeth et al.,2015). At the same time, a large number of competitors spawned by network capabilities and environment make it easy for customers to switch online vendors without high switching cost (Hee-Woong et al., 2013). Up to now, substantial studies have been aimed at finding ways to increase customer trust and solve the trust crisis, and also figured out there are still many limitations exist. From another aspect, it also proves the importance and eternal existence of trust issues.
Automatic Recommendation System
A recommendation system is a system of information filtering that studies user interests based on information from user's profiles or previous behavioral records, and forecast the user's preference or ratings for a given item as well as make a recommendations accordingly (John.O & John.D, 2004; Venkatesh et al., 2002). It alters the way businesses connect with users and strengthens the relations with users, is able to provide a personalized service, and helps buyers and sellers reduce the information overload they face (Bo & Izak, 2007). From the perspective of E-commerce, recommender system help users to search for the products of their preference and interest through the record of knowledge (Isinkaye, Folajimi, & Ojokoh, 2015). Usually, the optimal decision requires an in-depth evaluation of all available alternatives with complete information and substantial knowledge, but it’s nearly inaccessible to humans due to their limited memory and bounded rationality. Kabiawu, van Belle, and Adeyeye (2016) suggested that a reliable information source such as Information and Communication Technologies (ICTs) is an effective solution to improve the bounded rationality situation.
The user-recommender interaction is a mutual action between the recommended system and users. After login to the system, users get multiple recommended items helping the users to select a preferred item (Jingjing & Shawn, 2018). In general, there are two main types of recommendation strategies; content-based recommendation and collaborative filtering. The content-based recommendation normally relies on information such as actors, gender, producer, or director, etc., while collaborative filtering relies on the users’ profile to capture the users’ past rating histories (O'Donovan & Smyth, 2005). Several studies suggest that collaborative filtering is a possible solution like a trust model to improve predictive accuracy in recommendation because recommendations are based on the rating history from a suitable recommendation set(Bach et al., 2020; O'Donovan & Smyth, 2005).
Using a recommendation system is expected to improve the quality of consumers’ decision making. These systems use recommendation agents (RAs), software agents that provoke the consumers’ interest for products either implicitly or explicitly. However, research suggested that different types and characteristics of RAs exert differential influence on consumers’ decision efforts, decision quality, and trust (Xiao & Benbasat, 2007). Moreover, the providers’ capability plays a key role in building users’ trust in RA. For example, Xiao and Benbasat (2007) suggested that there are several other factors that moderate the effects of RA on consumers’ decision-making process. For example, they found that the effects of RA use and characteristics of RA on product choice, decision efforts, and decision quality are stronger for the multifaceted products. That means that if consumers have higher product expertise, their decision quality will be less likely affected by RAs. Alternatively, in the case of consumers’ higher risk perception of the product, the decision-making process is highly affected by the RA. This suggests that users’ evaluation of RA is dependent on many factors including, RA use, RA characteristics (such as input, design, output), and some other factors related to the user, product, and user-RA interaction.
In addition to the above, several studies have found that another key factor affecting the consumers’ trust in online environment is privacy (Yuanchun et al., 2010). Recommendation systems rely on the user’s profile to give recommendations as they work on the concept that users tend to purchase those products that they have purchased already. You Tube is a as a real life example, it usually offers the users only those videos that are similar or related to what they have already watched on their recommendation page. This is because that recommendation systems track users’ data and browsing history. These privacy issues increase the customers’ perceived risk and lower the trust in using recommendation system (Yuanchun et al., 2010).
The study of trust issues in the recommendation system is not a new topic. Many scholars have researched the topic from different perspectives to examine what can affect the users’ decisionmaking process based on a recommendation system (Xiao & Benbasat, 2007). However, the evolution in the e-commerce industry and upgrades of RS has added new features which have made it easier to use RS with more complicated back end operation and turned out to be standard on various platforms such as image social platforms, video platforms, and short video channels, like Tiktok, and the Facebook. A recent update in this regard is the feature of Social Media
Shopping that refers to companies’ direct selling points within the platforms by using content. It forms a great combination with RS on the basis of marketing (Sidharth Muralid &Lin, 2015). According to (y Monsuwé, Dellaert, & De Ruyter, 2004) consumers perceive human expertise and interaction more trustworthy in online shopping and it acts as a factor that increases trust in e-commerce.
Although the RS upgrades in terms of new features and types has offered many opportunities to the e-commerce retailers, it also brings new risks. Researchers are focusing to explore those risky areas. For example, Sylvain and Jacques have critically examined the comparison between the RS and other different information systems. They found that users perceive the recommendations of other consumers as more trustworthy than the recommendation received from RS (Sylvain & Jacques, 2004). The findings of this research provide part of the basis for inferring the hypothesis of this paper.
Influencer Marketing and curator system
Influencer Marketing
With the rapid evolution of social media networks over the last few years, influencer marketing has been evolved as a new marketing strategy. This is because today everyone has online access to market its products or services and influence the behavior of their followers. Celebrity endorsement was the original form of influencer marketing but in today’s digital world, social content creators with a niche audience can offer more value to the brands. These people have dedicated and engaged groups of followers on social media and are known as social media influencers (Chen & Shupei, 2018).
According to Berger (2016) influencers are being perceived as more credible believable and knowledgeable and they found that about 82% of consumers are likely to follow the influencers’ recommendations. Similarly, Johansen and Guldvik (2017) state that online reviews and friends recommending something in forums serve as influential marketing. While looking at how the items are being used in daily life, one tends to associate influencers with a certain type of people, normally a more influential crowd. Influencers use attractive content to show their love for product recommendations as they enjoy a similar status to celebrities and are considered more trustworthy.
Li, Xiong, Wang, Chen, and Xiong (2019) suggest that with the popularity of social media networks, exploiting the social relationship offers a reliable source to enhance the performance of the recommendation system. Therefore, social media influencers have the potential to improve the recommendation system by building trust relationships. In social media, the combination of the online social network and recommendation system has created many opportunities for businesses that consider the importance of influential marketing in marketing their products (Goanta & Spanakis, 2020).
Another reason for increased sales derived from influencer marketing is the halo effect created by the influencers through their content (Tapinfluence & Nielsen, 2016). Moreover, Influencer marketing is cost-effective than traditional marketing and can lead to marketing innovation as well. At the same time, consumers would continually have opportunities to review the contents even after an influencer marketing campaign is done, which bring an on-going effect made by the campaign for companies.
Curator system
As mentioned in the introduction part, this paper seeks to set up a new framework to simplify the existing model and make an easy comparison between the original RS and RS with social media influencers. This paper proposes a user recommendation system that prototypes preference for both users and the items they involve simultaneously. Curators are the individuals who gather and arrange existing content and enrich it with extra personal insights including comments, reviews, or ratings in different forms, such as a tweet, blog posts, or photos. They usually have a certain level of expertise or influence as an opinion leader (Jianling, Ziwei & James, 2020).
Curators can help the users to discover new items, new connections, and the new collection by human power rather than only provides product information pages by algorithm. In the curation platform, users act as curators who collect and organize content by reviews, tagging, or ratings. By receiving updates from whom they follow, they are exposed to interesting items and curation decisions. For example, a Spotify user may follow other users by tracking their listening activities which can influence their decisions. Research shows that the power of human curation can better serve as an important component of modern recommendation systems to link users to items. Moreover, the element of human power in the curated recommendation system enhances the transparency and trust of the users(Dragovic, Madrazo Azpiazu, & Pera, 2018; Wang, Zhu, & Caverlee, 2020). Using one the most famous e-commerce platform, called Xiaohongshu, also known as RED. In the Xiaohongshu community, users and celebrities can share their life, product reviews and travel guides.
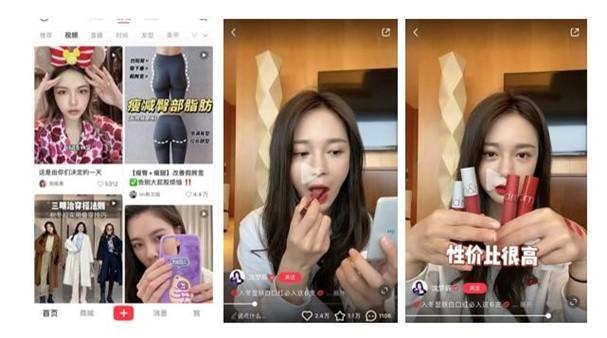
As can be seen in the picture above, the users are able to view the videos or blogs of other consumers or curators based on their previous browsing history or their followed channels on their the homepage.
Since the research of Sylvain & Jacques and a lot of other researches stated that users would have different perceptions towards expertise, other consumers, and celebrities. Therefore, two main types of the curator will be investigated in this paper, one is the curator that focuses on using human expertise, and another one is the influencer curator who focuses on using their popularity, to see how different the degree of users’ perception of trust in the recommendation has been affected.
Trust Model
Trust is a complicated topic as it is a subjective indicator, which is hard to measure. Rotter (1967) defines trust as “a generalized expectancy held by an individual that the word of another...can be relied on.” According to Komiak and Benbasat (2006), emotional trust and cognitive trust in recommendation agent significantly increase the customers’ attention to take help from the recommendation agent to make a purchase decision. However, they further found that emotional trust plays a more important role beyond cognitive trust in consumers’ attention to adopting RA. According to Komiak and Benbasat (2006), cognitive trust is defined as the rational expectations; a customer has about the capability of RA to provide useful product recommendations. It implies customers develop cognitive interest when they find valid and good reasons to trust. While emotional trust is a trusting behavior primarily motivated by a strong positive impact for the object such as sense of security or comfort (Komiak and Benbasat ,2006).
McKnight, Choudhury, and Kacmar (2002) have proposed a trust-building model for build-ing trust in e-commerce vendors. The model includes three perspectives; perceived website quality, perceived vendor reputation, and structural assurance i.e., consumers’ perception of web environment safety. According to this study, all three factors significantly influence the consumers’ trust in the web vendors. Particularly, the website reputation and quality are the critical factors that online vendors can use to overcome the negative perceptions and to build consumers' trust in the safety and security of the web environment. The findings of McKnight et al. (2002) have further extended by (Pavlou, 2003) who proposed the technology acceptance model (TAM) to explain that perceived usefulness and ease to use the website are key factors for e-commerce acceptance. This proposed model assimilates perceived risk and trust which are merged given the implied uncertainty of the online environment. Similar to McKnight et al. (2002) and McKnight et al. (2002), the trust in websites has been al-so supported by Sheng (2012) as a key driver to increase the customer trust in RA and RA’s recommendations. He further found that consumer participation in using RA can also increase the customer interest in RA which in turn can increase their intentions to buy items based on RA’s recommendations.
This study takes a trust-based, emotional approach to study Recommendation Agent adoption. Based on the trust literature and theory of reasoned action, this study critically examines the role of emotional trust in encouraging consumers to adopt RA in making purchasing decisions. The theoretical foundation of this study is drawn from the Theory of Reasoned Action (TRA). The relationship between emotional trust, cognitive trust, and purchase intention is well documented by the TRA framework (Fishbein & Ajzen, 1977).
Using TRA as a theoretical approach, the research model of this study illustrates the causal cycle from cognitive trust to emotional trust to use intention i.e., trusting intention to adopt RA for decision making. The existing literature considers the trust-related implications of both emotional factors i.e., institutional factors, and cognitive factors i.e, vendor-specific trustbuilding drivers (Komaik and Benbasat , 2006; McKnight et al. 2002). The vendor-specific factors include the reputation and quality of the website. The cognitive trust in integrity and competence of RA are the beliefs customer develop based on rational reasoning such as website quality and reputation. The institutional factors are the users’ perception of the online environment that serves as an attitude and intention towards the behavior of RA adoption. Thus, emotional trust as an attitude is an evaluative effect from relying on RA and any increase in cognitive trust in integrity and competence will increase customer’s emotional trust. Therefore, Trusting beliefs and trusting intention together means trust as has been stated by McKnight et al. (2002). Trust helps customers to overcome uncertainty and the risk perception.
On the other side, Komiak and Benbasat (2006) argued emotional trust is more important without which cognitive trust is inappropriate to examine how people make purchase decision. They argued that people’s cognition abilities are overstated due to rational choice perspectives. Moreover, the rational choice perspective allows little emotional and social influence on trust decisions. Emotional trust is defined as a trusting behavior derived from the positively strong effect for the trust object (Komaik and Benba-sat ,2006). Based on the literature, this study conceptualizes the emotional trust as an attitude towards adoption of RA as it is an evaluating effect for relying on RA. According to TRA, attitude is the major factor behind the person’s intention to perform a specific behavior. In the case of RA adoption, high cognitive trust in the competence of RA means that customers perceive that relying on recommendation agents will provide well-customized recommendation. This suggests that cognitive trust is interlinked with the emotional trust and any increase in emotional trust is derived by the cognitive trust in competence and integrity of RA. To simplify, a positive attitude towards purchasing behavior will lead to the purchase intention i,e., customers tend to adopt an RA when they will have a high level of emotional trust. Moreover, the way emotional trust complements the cognitive trust provides the clear understanding of trust intention and RA adoption. Therefore this study uses emotional trust i.e., trusting intention as a major driver to the adoption of recommendation agent.